일 | 월 | 화 | 수 | 목 | 금 | 토 |
---|---|---|---|---|---|---|
1 | ||||||
2 | 3 | 4 | 5 | 6 | 7 | 8 |
9 | 10 | 11 | 12 | 13 | 14 | 15 |
16 | 17 | 18 | 19 | 20 | 21 | 22 |
23 | 24 | 25 | 26 | 27 | 28 |
- YAI 8기
- YAI 10기
- Faster RCNN
- Fast RCNN
- Googlenet
- CNN
- YAI
- CS231n
- RCNN
- GaN
- PytorchZeroToAll
- 컴퓨터비전
- NLP #자연어 처리 #CS224N #연세대학교 인공지능학회
- 컴퓨터 비전
- nerf
- YAI 11기
- NLP
- 강화학습
- transformer
- 자연어처리
- cl
- YAI 9기
- VIT
- rl
- 3D
- CS224N
- cv
- 연세대학교 인공지능학회
- Perception 강의
- GAN #StyleCLIP #YAI 11기 #연세대학교 인공지능학회
- Today
- Total
목록연세대학교 인공지능학회 (46)
연세대 인공지능학회 YAI
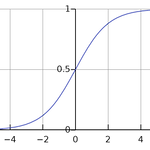
YAI 11기 조믿음님이 기초부원팀에서 작성한 글입니다 Study Material PyTorchZeroToAll Lecture 1 ~ 11 모두를 위한 딥러닝 시즌 2 Lab 1 ~ 6 Index What is Machine Learning? Linear Model Linear Regression Gradient Descent Back Propagation Chain Rule Review Logistic Regression Binary Prediction Sigmoid Function Binary Cross Entropy Loss Softmax Classifier Softmax Function PyTorch Basics Discussion What is Machine Learning/Deep Learni..
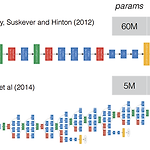
YAI 11기 최가윤님이 작성한 글입니다. [GoogLeNet] Going deeper with convolutions (2015 CVPR) Reference https://arxiv.org/pdf/1409.4842.pdf https://en.wikipedia.org/wiki/Gabor_filter [GoogLeNet (Going deeper with convolutions) 논문 리뷰]https://phil-baek.tistory.com/entry/3-GoogLeNet-Going-deeper-with-convolutions-%EB%85%BC%EB%AC%B8-%EB%A6%AC%EB%B7%B0 Abstract Improved utilization of the computing resources inside..
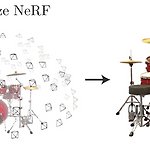
YAI 9기 박준영님이 나의야이아카데미아팀에서 작성한 글입니다. NeRF : Representing Scene as Neural Radiance Fields for View Synthesis [Mildenhall, Ben, et al. "Nerf: Representing scenes as neural radiance fields for view synthesis." Communications of the ACM 65.1 (2021): 99-106.](https://dl.acm.org/doi/abs/10.1145/3503250) Abstract 이 논문은 input으로 한정된 수의 3D scene을 획득, 이를 활용하여 continous volumetric scene function에 입각한 여러 방향에서..
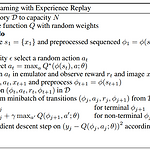
YAI 9기 박찬혁님이 논문구현팀에서 작성한 글입니다. 논문 Playing Atari with Deep Reinforcement Learning Playing Atari with Deep Reinforcement Learning 이번 논문에서는 강화학습을 위한 첫번째 딥러닝 모델인 DQN을 소개한다. 기존의 강화학습과 DNN을 결합시키기에는 몇가지 문제점이 있었다. 보통의 딥러닝 지도학습 task들은 라벨링 된 데이터들이 있었지만 강화학습에서는 이런 데이터들이 거의 없으며 노이즈가 많고 딜레이가 있는 reward를 통해 학습을 진행시켜야한다. 또한, 기존 딥러닝은 데이터간의 연관 관계가 없는 독립 관계라고 가정하고 진행하지만 강화학습의 경우 각 state들이 큰 연관성이 있다. 이 어려움들을 해결하고 강..
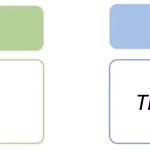
CS224N Lecture 15~18 * 10기 김준완님이 자연어 강의 팀에서 작성하신 리뷰입니다. Language Model Language Model은 Masked LM과, Standard LM 두가지로 나눌 수 있는데, 전자는 BERT류의 Deonising Auto Encoding이고, 후자는 기존의 조건부 확률 기반으로 다음에 올 단어를 예측하는 Auto Regressive 한 모델이다. 이런 예측된 값들은 make sense하지만 항상 factually correct하진 않다. 그 이유는 unseen facts, rare facts, model sensitivity때문이라고 할 수 있다. 여기서 sensitivity는 문맥상 같은의미의 문장이라도 모델은 사용된 단어가 다르면 다른 문장으로 인식할..
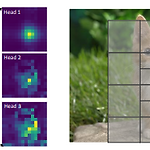
YAI 9기 김석님이 비전논문팀에서 작성한 글입니다. Focal Self-attention for Local-Global Interactions in Vision Transformers 0. Abstract 목적 Self attention을 통한 짧은 것에서부터 긴 단위까지 visual dependency를 모두 capture할 수 있도록 설계하면서도 quadratic computational overhead로 인한 resolution이 높은 task에 관해서 어려운 상황도 극복할 수 있어야 함 Method SoTA model의 경우 coarse-grain이나 fine-grained local attention을 적용하여 computational & memory cost와 성능을 개선하는 방식을 채택함 ..
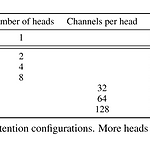
Diffusion Models Beat GANs on Image Synthesis ** YAI 9기 최성범님께서 DIffusion팀에서 작성한 글입니다. Abstract Generation model중 diffusion models이 SOTA를 달성 이를 위해 better architecture와 classifier guidance를 사용함 Classifier guidance는 classifier의 gradients를 사용하고, generated image의 diversity와 fidelity의 trade off 관계가 있음 1. Introduction GAN은 최근 FID, Inception Score, Precision metric으로 측정한 image generation task에서 SOTA를 달성..
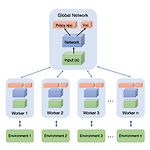
YAI 9기 이상민님이 강화학습 논문구현팀에서 작성한 글입니다 Asynchronous Methods for Deep Reinforcement Learning Asynchronous Methods for Deep Reinforcement Learning 🌎YAI 9기 이상민 논문소개 Asynchronous Methods for Deep Reinforcement Learning Simple and lightweight Deep Reinforcement Learning framework multi core CPU instead of GPU. Asynchronous gradient descent Parallel A3C Introduction Deep nueral networks가 Reinforcement Lea..