일 | 월 | 화 | 수 | 목 | 금 | 토 |
---|---|---|---|---|---|---|
1 | 2 | 3 | 4 | |||
5 | 6 | 7 | 8 | 9 | 10 | 11 |
12 | 13 | 14 | 15 | 16 | 17 | 18 |
19 | 20 | 21 | 22 | 23 | 24 | 25 |
26 | 27 | 28 | 29 | 30 | 31 |
- YAI 11기
- 컴퓨터 비전
- VIT
- RCNN
- CNN
- NLP
- 컴퓨터비전
- 연세대학교 인공지능학회
- Faster RCNN
- GaN
- nerf
- YAI
- 3D
- PytorchZeroToAll
- cv
- cl
- GAN #StyleCLIP #YAI 11기 #연세대학교 인공지능학회
- 강화학습
- CS231n
- YAI 8기
- transformer
- Googlenet
- rl
- YAI 9기
- Fast RCNN
- Perception 강의
- YAI 10기
- CS224N
- NLP #자연어 처리 #CS224N #연세대학교 인공지능학회
- 자연어처리
- Today
- Total
목록컴퓨터비전 : CV (24)
연세대 인공지능학회 YAI
CoCa : Contrastive Captioners are Image-Text Foundation Models https://arxiv.org/abs/2205.01917 CoCa: Contrastive Captioners are Image-Text Foundation Models Exploring large-scale pretrained foundation models is of significant interest in computer vision because these models can be quickly transferred to many downstream tasks. This paper presents Contrastive Captioner (CoCa), a minimalist design..
StyleGAN-NADA: CLIP-Guided Domain Adaptation of Image Generators https://arxiv.org/abs/2108.00946 StyleGAN-NADA: CLIP-Guided Domain Adaptation of Image Generators Can a generative model be trained to produce images from a specific domain, guided by a text prompt only, without seeing any image? In other words: can an image generator be trained "blindly"? Leveraging the semantic power of large sca..
StyleCLIP: Text-Driven Manipulation of StyleGAN Imagery https://arxiv.org/abs/2103.17249 StyleCLIP: Text-Driven Manipulation of StyleGAN Imagery Inspired by the ability of StyleGAN to generate highly realistic images in a variety of domains, much recent work has focused on understanding how to use the latent spaces of StyleGAN to manipulate generated and real images. However, discovering semanti..
U-Net: Convolutional Networks for Biomedical Image Segmentation ** YAI 10기 안정우님이 비전논문기초팀에서 작성한 글입니다. Abstact Present a network and training strategy that relies on the strong use of data augmentation Use available annotated samples more efficiently The architecture consists of a Contracting path and a symmetric Expanding path Contracting path Captures content Expanding path Enables precise local..
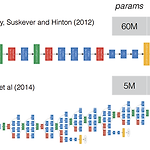
YAI 11기 최가윤님이 작성한 글입니다. [GoogLeNet] Going deeper with convolutions (2015 CVPR) Reference https://arxiv.org/pdf/1409.4842.pdf https://en.wikipedia.org/wiki/Gabor_filter [GoogLeNet (Going deeper with convolutions) 논문 리뷰]https://phil-baek.tistory.com/entry/3-GoogLeNet-Going-deeper-with-convolutions-%EB%85%BC%EB%AC%B8-%EB%A6%AC%EB%B7%B0 Abstract Improved utilization of the computing resources inside..
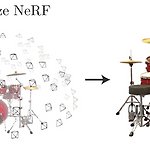
YAI 9기 박준영님이 나의야이아카데미아팀에서 작성한 글입니다. NeRF : Representing Scene as Neural Radiance Fields for View Synthesis [Mildenhall, Ben, et al. "Nerf: Representing scenes as neural radiance fields for view synthesis." Communications of the ACM 65.1 (2021): 99-106.](https://dl.acm.org/doi/abs/10.1145/3503250) Abstract 이 논문은 input으로 한정된 수의 3D scene을 획득, 이를 활용하여 continous volumetric scene function에 입각한 여러 방향에서..
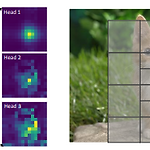
YAI 9기 김석님이 비전논문팀에서 작성한 글입니다. Focal Self-attention for Local-Global Interactions in Vision Transformers 0. Abstract 목적 Self attention을 통한 짧은 것에서부터 긴 단위까지 visual dependency를 모두 capture할 수 있도록 설계하면서도 quadratic computational overhead로 인한 resolution이 높은 task에 관해서 어려운 상황도 극복할 수 있어야 함 Method SoTA model의 경우 coarse-grain이나 fine-grained local attention을 적용하여 computational & memory cost와 성능을 개선하는 방식을 채택함 ..
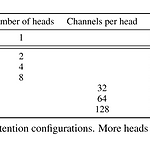
Diffusion Models Beat GANs on Image Synthesis ** YAI 9기 최성범님께서 DIffusion팀에서 작성한 글입니다. Abstract Generation model중 diffusion models이 SOTA를 달성 이를 위해 better architecture와 classifier guidance를 사용함 Classifier guidance는 classifier의 gradients를 사용하고, generated image의 diversity와 fidelity의 trade off 관계가 있음 1. Introduction GAN은 최근 FID, Inception Score, Precision metric으로 측정한 image generation task에서 SOTA를 달성..